TGVP Report - Enterprise GenAI Market Landscape and Potential Investment

Enterprise GenAI Market Landscape and Potential Investment Opportunities
Written by Saksham Bhandari (Intern from Kellogg School of Management / SF Immersion Program Winter 2025)
Enterprise GenAI Market Map
At a 10,000 feet level, the overall Enterprise GenAI tech landscape can be classified into the following 3 categories:
Infrastructure Layer: Includes the foundational layer of technologies (hardware + software) that enable development, training, and deployment of GenAI models / apps.
Application Layer: Tools / platforms that are built on top of Infrastructure layer and leverage GenAI foundational models to create practical, user-facing applications and solutions. The primary focus here is on making AI capabilities accessible and useful for businesses
AI Enabled Services: Firms leveraging AI tech (3rd party or self-built) to provide highly customizable services to end clients – helping in the automation of routine tasks. Such businesses can scale more non-linearly compared to traditional service businesses by using AI agents at the backend.

Categories of Interests

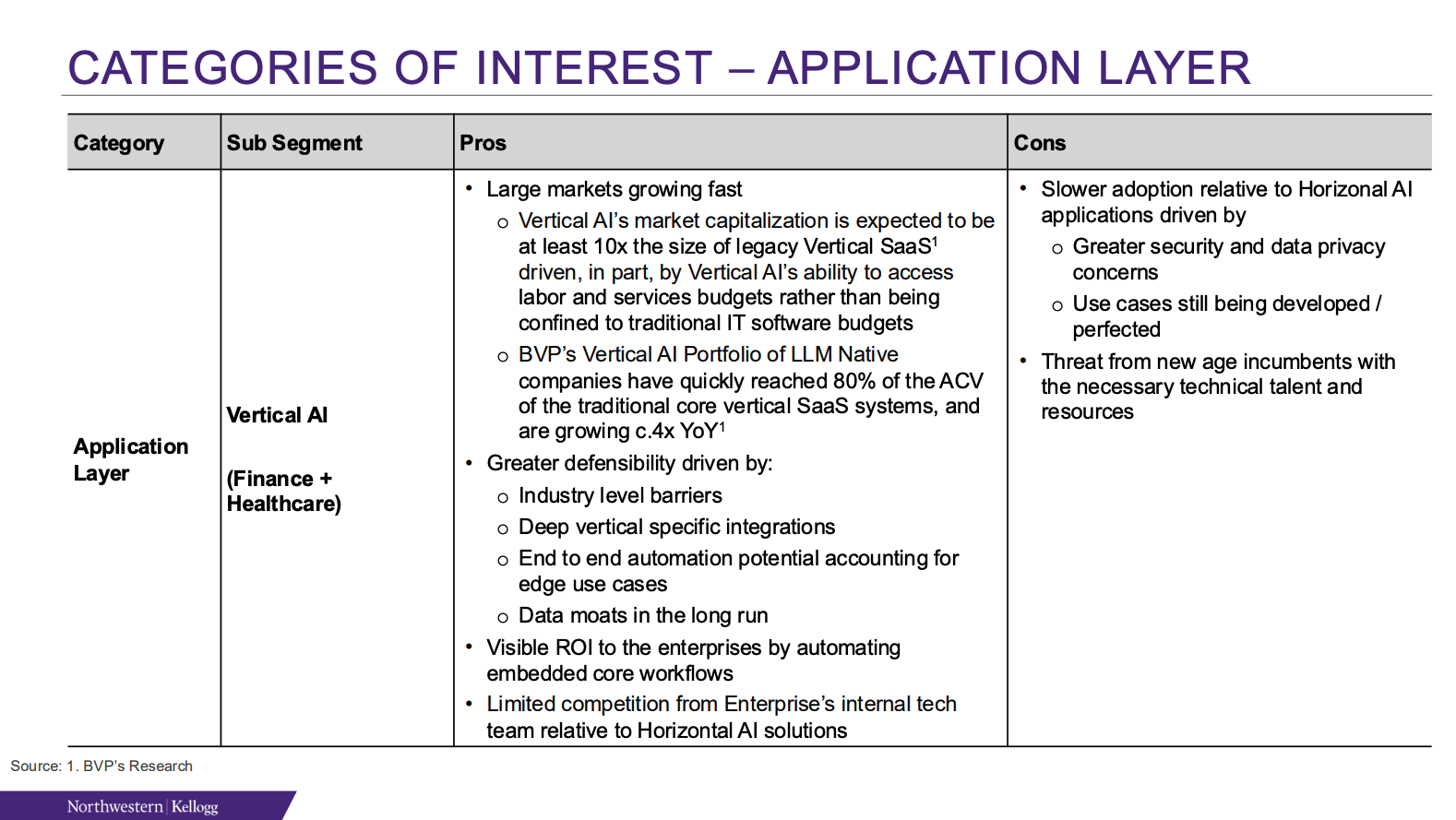
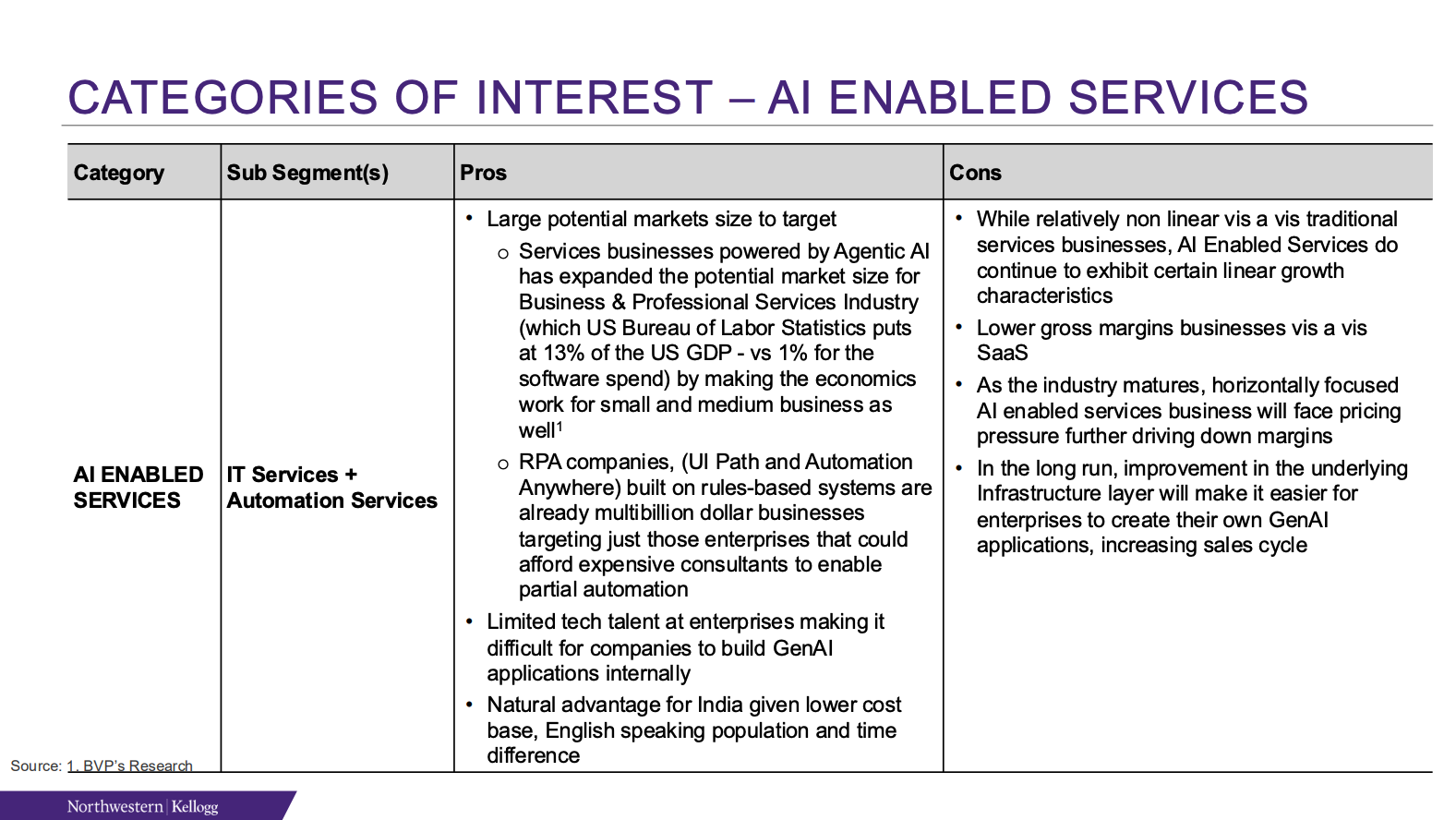
Application Layer – Deep Dive
As enterprises start moving from experimentation to execution on GenAI, the Application Layer is expected to grow significantly and therefore offers the maximum growth potential
- Between 2023 and 2024, the total spend on the AI Application layer has increased from $600M to $ 4.6B, growing c.8x in 1 year
- The spend on the application layer is only expected to increase as most companies are still in the early stages of adoption, with only a few use cases in production, while a 33% of them are still being prototyped and evaluated
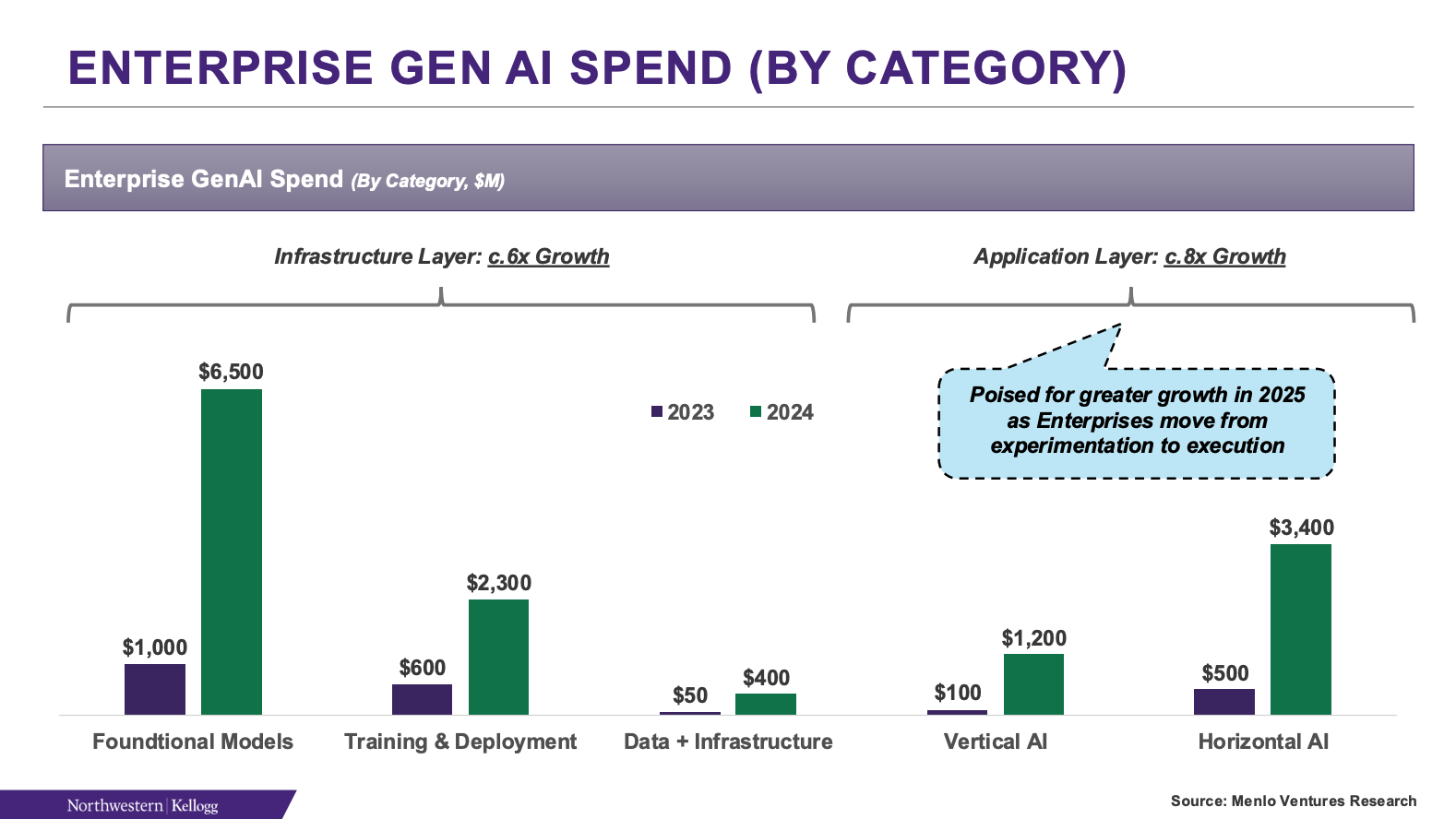
BFSI is well positioned for Agentic AIdisruption
- Growing Adopter of Agentic AI Solutions: Data from Microsoft highlights that the BFSI as one of the leaders in AI adoption based on consumption revenue data for cloud services on the Azure platform using foundation models.
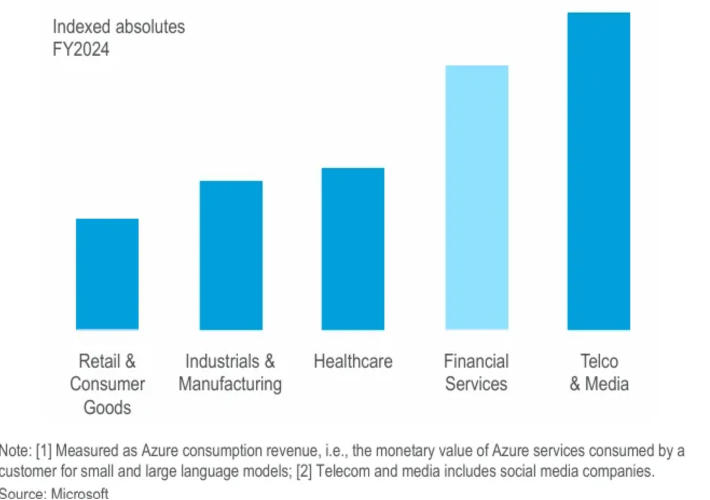
- Large Potential Market Size: Large market with several small, medium and large size clients across geographies and categories (Banks, Payment Companies, Insurance, Wealth Advisory, Brokerages, etc.). Historically too banks have been the biggest spender on technology (outside of the tech industry)
- Strong Regulatory Moat: Barrier to entry in the form of licensure requirements and other regulatory hurdles
- Text and Quantitative Heavy Work Nature: Text-and quantitative-heavy work have the highest propensity for automation under the agentic AI paradigm
BFSI - Agentic AI Use Cases

BFSI clients need enormous headcount to review a lot more alerts when 95% is wasted effort. Focusing on far better data collection, labelling, and then ML to identify true positives can save massive amounts of time and money for BFSI clients.
- The average U.S. firm today spends between 1.3-3.3% of its total wage bill on compliance. Basis Greylock, majority of enterprises spend c.$30B annually on human compliance analysts
- Within BFSI, basis a 2020 survey, 1/3rd of firms had allocated at least 5% of their annual budgets to regulatory compliance. The reason for high is the manual nature of current compliance workflows, coupled with the continuously evolving and complex nature of regulation (e.g., the US has several different regulators for different types of financial products, sometimes at the state level)
- Banking and insurance regulations are super cumbersome and span tens of thousands of pages making it difficult for humans to keep up with. For Instance: Over the last 50 years, the number of restrictive words in the U.S. Code of Federal Regulations has grown from 400,000 to over 1 million
Rubrik for Series A/B Deal Evaluation
